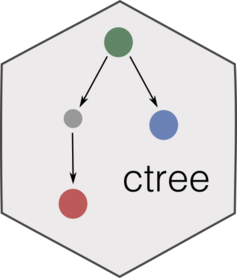
Construct a `ctree` clone tree with known structure.
ctree.Rd
This constructor creates an object of class `'ctree'`, which represents a clone tree. The tree is created from a set of clusters computed for a patient, usually with methods that carry out tumour subclonal deconvolution routines on bulk DNA sequencing data.
To create a tree a list of drivers can be provided to be annotated to an input set of CCF clusters. There are a minimum amount of information and formatting fields that are required for tree construction to operate successfully. Please refer to the package vignette and the provided input datasets for more instructions.
Usage
ctree(
CCF_clusters,
drivers,
samples,
patient,
M,
score,
annotation = paste0("Clone tree for patient ", patient)
)
Arguments
- CCF_clusters
Clusters of Cancer Cell Fractions available in the data of this patient. See the package vignette to see the format in which this should be specified.
- drivers
A list of driver events that should be annotated to each one of the input clusters contained in the `CCF_clusters` parameter. See the package vignette to see the format in which this should be specified.
- samples
A vector of samples names (e.g., the biopsies sequenced for this patient).
- patient
A string id that represent this patient.
- M
The adjacency matrix defined to connect all the nodes of this tree.
- score
A scalar score that can be associated to this tree.
- annotation
Any string annotation that one wants to add to this `ctree`. This will be used by some of the plotting functions that display `ctree` objects.
See also
This function requires the input tree to be specified in the
format of an adjacency matrix; plese see function ctrees
if you
need to create de novo also the adjacency matrices that fit your data.
Examples
data('ctree_input')
x = ctrees(
ctree_input$CCF_clusters,
ctree_input$drivers,
ctree_input$samples,
ctree_input$patient,
ctree_input$sspace.cutoff,
ctree_input$n.sampling,
ctree_input$store.max
)
#> [ ctree ~ clone trees generator for CUK12345 ]
#>
#> # A tibble: 7 × 7
#> cluster nMuts is.driver is.clonal R1 R2 R3
#> <chr> <int> <lgl> <lgl> <dbl> <dbl> <dbl>
#> 1 1 72 TRUE FALSE 0 0.92 0
#> 2 2 69 TRUE TRUE 0.99 0.98 0.99
#> 3 3 48 FALSE FALSE 0 0 0.49
#> 4 4 29 FALSE FALSE 0.01 0.01 0.93
#> 5 5 24 TRUE FALSE 0.78 0 0
#> 6 6 23 TRUE FALSE 0.98 0.03 0.98
#> 7 7 15 FALSE FALSE 0 0.41 0
#>
#> ✔ Trees per region 1, 3, 1
#> ℹ Total 3 tree structures - search is exahustive
#>
#> ── Ranking trees
#> ✔ 3 trees with non-zero score, storing 3
x = x[[1]]
# Adj matrix inside of the objects, we remove the GL
# entry that is added as fake root by ctree
M = x$adj_mat
M = M[rownames(M) != 'GL', colnames(M) != 'GL']
print(M)
#> 2 1 6 4 7 5 3
#> 2 0 1 1 0 0 0 0
#> 1 0 0 0 0 1 0 0
#> 6 0 0 0 1 0 1 0
#> 4 0 0 0 0 0 0 1
#> 7 0 0 0 0 0 0 0
#> 5 0 0 0 0 0 0 0
#> 3 0 0 0 0 0 0 0
# Manual construction
y = ctree(
ctree_input$CCF_clusters,
ctree_input$drivers,
ctree_input$samples,
ctree_input$patient,
M,
score = 123456,
annotation = paste0("Some clone tree")
)
# The same
print(x)
#> [ ctree - ctree rank 1/3 for CUK12345 ]
#>
#> # A tibble: 7 × 7
#> cluster nMuts is.driver is.clonal R1 R2 R3
#> <chr> <int> <lgl> <lgl> <dbl> <dbl> <dbl>
#> 1 1 72 TRUE FALSE 0 0.92 0
#> 2 2 69 TRUE TRUE 0.99 0.98 0.99
#> 3 3 48 FALSE FALSE 0 0 0.49
#> 4 4 29 FALSE FALSE 0.01 0.01 0.93
#> 5 5 24 TRUE FALSE 0.78 0 0
#> 6 6 23 TRUE FALSE 0.98 0.03 0.98
#> 7 7 15 FALSE FALSE 0 0.41 0
#>
#> Tree shape (drivers annotated)
#>
#> \-GL
#> \-2 :: MET, TERT
#> |-1 :: RB1, IKZF1, KRAS
#> | \-7
#> \-6 :: EP300
#> |-4
#> | \-3
#> \-5 :: NF1
#>
#> Information transfer
#>
#> MET ---> RB1
#> MET ---> IKZF1
#> MET ---> KRAS
#> TERT ---> RB1
#> TERT ---> IKZF1
#> TERT ---> KRAS
#> GL ---> MET
#> GL ---> TERT
#> EP300 ---> NF1
#> MET ---> EP300
#> TERT ---> EP300
#>
#> Tree score 0.6
#>
print(y)
#> [ ctree - Some clone tree ]
#>
#> # A tibble: 7 × 7
#> cluster nMuts is.driver is.clonal R1 R2 R3
#> <chr> <int> <lgl> <lgl> <dbl> <dbl> <dbl>
#> 1 1 72 TRUE FALSE 0 0.92 0
#> 2 2 69 TRUE TRUE 0.99 0.98 0.99
#> 3 3 48 FALSE FALSE 0 0 0.49
#> 4 4 29 FALSE FALSE 0.01 0.01 0.93
#> 5 5 24 TRUE FALSE 0.78 0 0
#> 6 6 23 TRUE FALSE 0.98 0.03 0.98
#> 7 7 15 FALSE FALSE 0 0.41 0
#>
#> Tree shape (drivers annotated)
#>
#> \-GL
#> \-2 :: MET, TERT
#> |-1 :: RB1, IKZF1, KRAS
#> | \-7
#> \-6 :: EP300
#> |-4
#> | \-3
#> \-5 :: NF1
#>
#> Information transfer
#>
#> MET ---> RB1
#> MET ---> IKZF1
#> MET ---> KRAS
#> TERT ---> RB1
#> TERT ---> IKZF1
#> TERT ---> KRAS
#> GL ---> MET
#> GL ---> TERT
#> EP300 ---> NF1
#> MET ---> EP300
#> TERT ---> EP300
#>
#> Tree score 123456
#>